Atmospheric inversion of greenhouse gas fluxes
Introduction
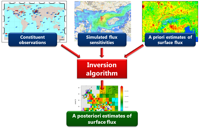
Regional scale
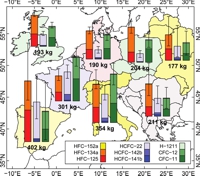
On the regional scale we use backward simulations of the Lagrangian Particle Dispersion Model FLEXPART to obtain flux sensitivities for limited areas. These describe the sensitivity towards surface fluxes within the given domain, whereas surface fluxes outside the domain are not explicitly treated but are taken as part of the baseline concentration entering the domain.
We implemented two regional scale inversion systems: a Bayesian inversion and an extended Kalman Filter inversion. In the Bayesian approach a cost function is optimised that contains a term penalising the misfit between model and observations and a term penalising deviations from the a priori state. The weighting between these two terms is controlled by two uncertainty covariance matrices that give the combined uncertainty of the model and the observation and an estimate of the a priori uncertainty. Using covariance matrices allows including spatiotemporal correlations in the involved uncertainties, which can further guide the inversion algorithm to find a realistic solution to the posed problem. In the Bayesian approach observations form different times and locations are used in the same optimisation step to obtain the a posteriori fluxes. In addition, to the surface fluxes other parameters, like the baseline concentrations at different times, can be part of the optimisation problem. A typical application of the Bayesian inversion technique is to the estimation of European scale halocarbon emissions by using the observations from the AGAGE stations Jungfraujoch, Mace Head and Monte Cimone (Keller et al., 2011, 2012). Similar analyses were done for north-eastern China (An et al., 2012, Vollmer et al., 2009).
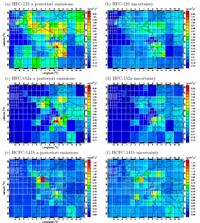
Global scale
On the global scale we use an adapted version of FLEXPART in a forward mode to continuously simulate atmospheric concentrations (Henne et al., 2013). In this configuration the whole atmosphere is filled with model particles that follow the atmospheric flow and are allowed to interact with the surface to take up emissions. The model also includes first order OH chemistry and, hence, is suited for the description of atmospheric methane. Each model particle is allowed to carry a large number of tracers. In this way we simulate concentrations for different types of emissions, different emission regions but, most importantly, emission times. The latter information together with the fact that, thanks to the first order chemistry, simulated concentrations linearly depend on emissions, allows us to construct the required flux sensitivities from the model output. We apply a Kalman smoother technique (Bruhwiler et al., 2005) to derive a posteriori flux estimates for the different emission types as a function of time. Currently the transport model and the inversion technique are applied to derive methane surface fluxes for the period 1990 to 2012 in the project MAIOLICA-II.
References:
- Brunner, D., Arnold, T., Henne, S., Manning, A., Thompson, R. L., Maione, M., O'Doherty, S., and Reimann, S.: Comparison of four inverse modelling systems applied to the estimation of HFC-125, HFC-134a and SF6 emissions over Europe, Atmos. Chem. Phys. Discuss., doi:10.5194/acp-2017-19, in review, 2017.
- Henne, S., Brunner, D., Oney, B., Leuenberger, M., Eugster, W., Bamberger, I., Meinhardt, F., Steinbacher, M., and Emmenegger, L.: Validation of the Swiss methane emission inventory by atmospheric observations and inverse modelling, Atmos. Chem. Phys., 16, 3683-3710, doi:10.5194/acp-16-3683-2016, 2016.
- An, X., Henne, S., Yao, B., Vollmer, M., Zhou, L., and Li, Y.: Estimating emissions of HCFC-22 and CFC-11 in China by atmospheric observations and inverse modeling, SCIENCE CHINA Chemistry, 55, 2233-2241, doi: 10.1007/s11426-012-4624-8, 2012.
- Bruhwiler, L. M. P., Michalak, A. M., Peters, W., Baker, D. F., and Tans, P.: An improved Kalman Smoother for atmospheric inversions, Atmos. Chem. Phys., 5, 2691-2702, doi: 10.5194/acp-5-2691-2005, 2005.
- Brunner, D., Henne, S., Keller, C. A., Reimann, S., Vollmer, M. K., O'Doherty, S., and Maione, M.: An extended Kalman-filter for regional scale inverse emission estimation, Atmos. Chem. Phys., 12, 3455-3478, doi: 10.5194/acp-12-3455-2012, 2012.
- Henne, S., Schnadt Poberaj, C., Reimann, S., and Brunner, D.: Global-scale tropospheric Lagrangian particle models with linear chemistry, in: Lagrangian Modeling of the Atmosphere, edited by: Lin, J. C., Gerbig, C., Brunner, D., Stohl, A., Luhar, A., and Webley, P., Geophysical Monographs, AGU, Washington, DC, 235-250, 2013.
- Keller, C. A., Hill, M., Vollmer, M. K., Henne, S., Brunner, D., Reimann, S., O'Doherty, S., Arduini, J., Maione, M., Ferenczi, Z., Haszpra, L., Manning, A. J., and Peter, T.: European Emissions of Halogenated Greenhouse Gases Inferred from Atmospheric Measurements, Environ. Sci. Technol., 46, 217-225, doi: 10.1021/es202453j, 2012.
- Keller, C. A., Brunner, D., Henne, S., Vollmer, M. K., O'Doherty, S., and Reimann, S.: Evidence for under-reported western European emissions of the potent greenhouse gas HFC-23, Geophys. Res. Lett., 38, L15808, doi: 10.1029/2011GL047976, 2011.
- Vollmer, M. K., Zhou, L., Greally, B. R., Henne, S., Yao, B., Reimann, S., Stordal, F., Cunnold, D. M., Zhang, X., Maione, M., Zhang, F., Huang, J., and Simmonds, P. G.: Emissions of ozone-depleting halocarbons from China, Geophys. Res. Lett., 36, L15823, doi: 10.1029/2009GL038659, 2009.
-
Share